Understanding the Importance of Machine Learning Data Labeling
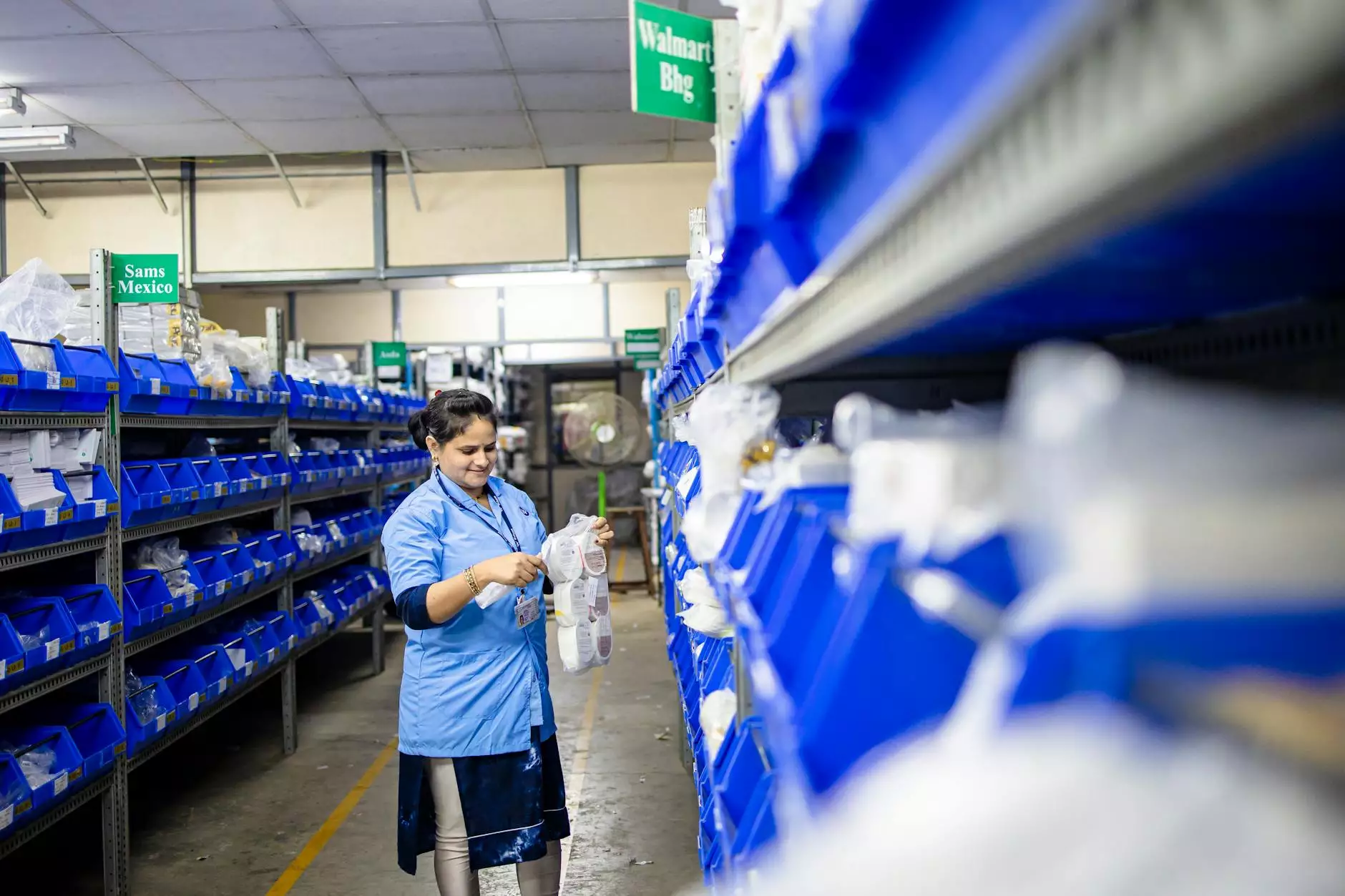
In the rapidly evolving landscape of technology, machine learning has emerged as a revolutionary tool, transforming the way businesses operate across various industries. Central to the success of machine learning algorithms is the critical process of data labeling. This article delves deep into the significance of machine learning data labeling, the techniques involved, and how it empowers businesses to leverage their data effectively.
The Foundation of Machine Learning: Data Labeling
Machine learning algorithms learn from data, and this data must be meticulously labeled to ensure high accuracy in the model's predictions. Data labeling involves the classification or tagging of information in datasets to define attributes, categories, or values in terms machine learning models can understand.
What is Machine Learning Data Labeling?
Machine learning data labeling is the process of annotating training data with meaningful tags that allow machine learning algorithms to learn from the data effectively. Each label represents a specific feature or category that helps the algorithm understand the context of the information it processes.
Types of Data Labeling
- Image Labeling: This involves tagging images with keywords or bounding boxes to identify objects within the images.
- Text Labeling: Text documents are annotated with labels to indicate topics, sentiments, or other relevant attributes.
- Audio Labeling: Sound files can be labeled to identify various types of sounds or spoken words.
- Video Labeling: Videos can be annotated with labels that describe the actions taking place at different timestamps.
Why is Machine Learning Data Labeling Essential?
The effectiveness of any machine learning model largely depends on the quality of the data it trains on. Here are key reasons why data labeling is essential:
1. Enhanced Model Accuracy
Accurate labeling leads to better understanding and hence better predictions by the AI model. When the data is tagged correctly, it aligns closely with the ground truth, allowing algorithms to make informed decisions based on that data.
2. Improved Training Speed
Properly labeled datasets can accelerate the training process. When data is clear and well-structured, learning algorithms require fewer iterations to achieve high accuracy rates.
3. Facilitating Complex Problem Solving
Data labeling is fundamental when dealing with complex problems like self-driving cars or facial recognition systems. In such cases, accurate data labeling allows for nuanced understanding and handling of intricate scenarios.
4. Continuous Learning Opportunities
Incorporating labeled data into machine learning models allows them to learn continuously. As more labeled data becomes available, models can refine their predictions and improve their performance over time.
Challenges in Machine Learning Data Labeling
While the advantages of data labeling are clear, the process comes with its own set of challenges:
1. Scalability Issues
As businesses grow and accumulate more data, the demand for data labeling can quickly outstrip internal capabilities. Manual labeling can be time-consuming and labor-intensive.
2. Subjectivity in Labels
Different labelers may interpret data differently, leading to inconsistencies in the labeling process. This subjectivity can introduce bias and undermine the quality of the dataset.
3. Cost Concerns
High-quality data labeling can be expensive, particularly if outsourced to specialized firms. Businesses must balance the need for accurate labels with budget constraints.
Keymakr: Your Partner in Data Labeling
At Keymakr.com, we understand the intricacies of machine learning data labeling and provide tailored solutions to meet your business needs. Our experienced team utilizes advanced technologies and methodologies to ensure accurate and efficient data labeling.
Our Services Include:
- Custom Data Labeling: We provide bespoke labeling services that cater to your specific requirements, whether it’s images, audio, text, or video.
- Quality Assurance: Each labeled dataset undergoes rigorous quality checks to maintain the highest standards of accuracy.
- Scalable Solutions: We have the resources to handle projects of any size, ensuring that your data labeling needs are met promptly.
- Expert Support: Our team of experts is always on hand to provide assistance and insights throughout the data labeling process, ensuring optimal results.
Best Practices for Effective Machine Learning Data Labeling
To ensure the highest quality in your machine learning projects, consider implementing the following best practices in data labeling:
1. Define Clear Guidelines
Establish comprehensive labeling guidelines that clearly define the labeling process. This helps in maintaining uniformity and reduces discrepancies among different labelers.
2. Use Multiple Labelers
Utilizing multiple labelers for the same dataset can help confront biases and ensure consistency. Dispute resolution mechanisms should be established for conflicting labels.
3. Regularly Validate Data
Routine validation of the labeled data should be conducted to ensure ongoing accuracy and quality. This can involve periodic checks and retraining of staff when necessary.
4. Invest in Automation
Consider using AI-assisted tools to automate parts of the labeling process. This can significantly enhance efficiency and consistency while reducing costs.
The Future of Machine Learning Data Labeling
As machine learning technology continues to evolve, the processes around data labeling will also undergo significant transformation. Here are some anticipated trends:
1. Increased Use of AI in Labeling
AI itself will play an increasingly pivotal role in the data labeling process, as algorithms become capable of automatically annotating data with greater accuracy.
2. Crowdsourced Data Labeling
Crowdsourcing will become a more prominent approach, leveraging the power of global contributors to label data efficiently and cost-effectively.
3. Focus on Privacy and Ethical Standards
With growing concerns over data privacy and ethics, businesses will need to prioritize ethical labeling standards to guide how data is handled.
Conclusion
The role of machine learning data labeling in the development of AI projects cannot be overstated. As a foundational step that directly impacts the performance and accuracy of machine learning models, effective data labeling is crucial. By partnering with experts like Keymakr, businesses can ensure the quality of their data labeling efforts, enabling them to harness the full potential of their data and drive their success forward.
Invest in your future with Keymakr and experience the benefits of professional data labeling today. Let's collaborate to elevate your machine learning projects to extraordinary heights!